Empirical Dynamic Modeling toward mechanistic understanding and forecasting complex ecosystems
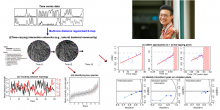
chih-hao hsieh
Institute of Oceanography, National Taiwan University, Taiwan
Link to seminar/ https://umontpellier-fr.zoom.us/webinar/register/WN_VaS9gkSrTPW7ZenXcM1AKg
Mechanistic understanding and forecasting are important for effective policy and management rec-ommendations for ecosystems. However, these tasks are challenging, because real world is complex, where correlation does not necessarily imply causation and interaction is not static. Here, I present a time-series analytical framework, known as Empirical Dynamic Modeling (EDM). EDM enables detecting causality among interacting components in nonlinear dynamical systems, constructing time-varying interaction networks, forecasting effects of external forcing, and serving as early warning signal for critical transition. I will introduce the methods and demonstrate the efficacy of EDM in various systems.
Grziwotz F., C. W. Chang, V. Dakos, E. H. van Nes, M. Schwarzländer, O. Kamps, M. Heßler, I. T. Tokuda, A. Telschow, and C. H. Hsieh. (2023) Anticipating the occurrence and type of critical transitions. Science Ad-vances. 9: eabq4558.
Chang, C. W., T. Miki, M. Ushio, P. J. Ke, H. P. Lu, F. K. Shiah, C. H. Hsieh (2021) Reconstructing large inter-action networks from empirical time series data. Ecology Letters. 24: 2763– 2774.
Sugihara, G., R. May, H. Ye, C. H. Hsieh, E. Deyle, M. Fogarty, and S. Munch (2012) Detecting causality in complex ecosystems. Science 338: 496-500.
Watch previous seminars on our YouTube channel: https://www.youtube.com/channel/UCrX4IsZ8WIFcDa0ZmC7rcQg